Unusual
calms tell of coming storms
By
Kimberly Patch,
Technology Research News
It seems that humans have always been trying
to predict the behavior of complicated systems like the weather and the
stock markets. It isn't easy.
Researchers from Oxford University have found a pattern in the way a large
entity like the stock market acts just before it undergoes a major change.
The finding is a step toward better predicting big changes in complicated
systems ranging from the stock market to computer networks, according
to the research.
When a population of agents competes for a limited resource, the overall
behavior of the system is predictable up to a point. In network
or commuter traffic, the agents are data packets or cars competing
for bandwidth or road space; in the stock market, the agents are traders
who are competing for monetary gain. The overall behavior is traffic speed
or market prices.
The researchers found that the level of predictability goes up just before
a large change. "Strategy selection amongst agents seems to converge --
it's kind of a crowd effect," said Neil Johnson, a lecturer in physics
at Oxford University. "Without communicating, or even knowing the existence
of each other, agents begin to lock into a particular behavior" just before
a large change, he said.
The finding is important because these large changes have strong effects
in financial, sociological and ecological systems, said Johnson. "These
are what upset the system and dictate what happens to it for a long time
thereafter," he said.
Although many big changes seem to happen randomly and almost without cause,
large systems like the stock market do contain a certain internal flow,
said Johnson. The market produces its own dynamics in the absence of significant
news, which is most of the time, he said. "So we wondered if the answer
to whether a large change is coming up is somehow already encoded in the
makeup of the system," he said.
Predictability also has to do with how stable a system is, said Johnson.
"Take, for example, commuter traffic. Usually everyone drives differently,
at slightly different speeds and with slightly different strategies --
this gives the traffic flow some kind of stability. If everyone is driving
at the same speed, in the same way, with the same distance between, then
this is unstable," he said. This is because if something happens to one
car, it is more likely to cause a chain reaction. "[If] something appears
on the road, it will cause a massive disruption," he said.
To find out if it is possible to predict a large change by looking at
the dynamics of a system before the change, the researchers simulated
a complicated system using a swarm of software agents. "We tried to predict
the system using something that [resembled] it," Johnson said.
The simulation used a population of software agents competing for limited
resources in a sort of musical chairs game that had less than half as
many chairs as agents.
The model roughly correlates to a buyer in a financial market who may
get a better price if more people are selling than buying, or a driver
who may have the quicker journey by choosing the route with less traffic,
according to Johnson.
In this model, the level of predictability in the system increased before
a large change, showing that the change was a consequence of the global
state of the system, and not something simply triggered by an isolated,
random event, said Johnson.
Usually a system rolls along with only reasonably small changes like the
daily changes in the stock market or the traffic speed in the usual nightly
commute home from the office, said Johnson. During these periods, changes
are almost random, he said. "If you want to predict whether the stock
will go up or down or whether one lane or another on the highway will
be best, than you might as well flip a coin."
This is because the internal forces in the system are finely balanced.
There's a large force created by traders who want to buy, but an almost
equal and opposite force created by traders who want to sell, he said.
"These changes are essentially random and hence unpredictable," he said.
When the forces become unbalanced, an avalanche-like effect happens "whereby
small glimpses of a pattern momentarily emerge and, by chance, become
amplified," said Johnson. It is during this amplification period that
the predictability of the system grows. "The agents start taking up definite
positions -- the two opposing forces are now momentarily out of balance.
After about 10 hours the imbalance begins to show itself as a definite
trend" in market prices, Johnson said.
The research is solid and makes sense within the researchers' model, but
whether it can be readily applied to real markets is debatable, said Kirill
Ilinski, a quantitative analyst in the equity derivatives department at
J.P. Morgan Chase in London.
"Within the model it's a natural result," said Ilinski. "What I would
really be very cautious about is their claim that this model actually
represents a real market. Directional moves are extremely difficult to
predict, especially in [markets that are] open 24 hours a day," he said.
This is because the stock market is a "many-phased thing," that is very
difficult to model, Ilinski said. "You have a huge number of players,
it's high-volume, very small margins. It's a very, very efficient market."
The researchers' next step is to focus on why the large changes happen,
said Johnson. "We can identify the buildup to these extreme events, but
what initiates them originally and how far back can we see one coming?"
The researchers are also working to relate the large-changes model to
real stock market data, said Johnson.
The research could eventually be applied to risk management, optimizing
traffic and regulating trading systems, Johnson said. For instance, the
best way to regulate trading systems or manage information networks is
to decrease the likelihood of a large, disruptive event, he said. The
question is, "how do you control such a system? Is it best to interfere
[with] each agent, or is there some tweaking you can do globally which
works just as well, or should you just leave the system to organize itself?"
The current version of of the software is aimed at exploring and testing
these concepts, but software aimed at applying them to real world problems
could be practical in two years, said Johnson.
Johnson's research colleagues were David Lamper and Sam Howison of Oxford
University. The research was funded by Oxford University.
Timeline: 2 years
Funding: University
TRN Categories: Applied Computing
Story Type: News
Related Elements: Technical paper, "Predictability of Large
Future Changes in a Competitive Evolving Population," posted on the Los
Alamos e-print archive at http://arXiv.org/abs/cond-mat/0105258.
Advertisements:
|
August
22/29, 2001
Page
One
Nets mimic quantum physics
Teamed filters catch
more spam
Software eases
remote robot control
Ion beams mold tiny holes
Unusual calms
tell of coming storms
News:
Research News Roundup
Research Watch blog
Features:
View from the High Ground Q&A
How It Works
RSS Feeds:
News | Blog
| Books 
Ad links:
Buy an ad link
Advertisements:
|
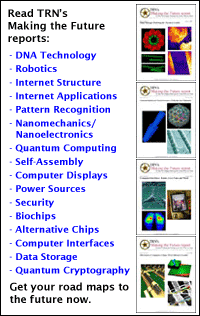
|
|
|