Rating
systems put privacy at risk
By
Ted Smalley Bowen,
Technology Research News
The Internet has given us new ways of carrying
out activities as diverse as shopping and political agitation, and many
of these new modes share a strong dependency on the medium’s shaky guarantees
of privacy and anonymity. This uncertainty has led to a variation on the
trap of guilt by association: the threat of exposure by indirect association
The chance you take when you use a Web
recommender is typical of this new jeopardy, which researchers at three
U.S. universities have quantified into basic equations of risk and benefit.
A Web recommender, or recommendation system, is a consumer rating system
popular with online buyers of books, movies, and other items whose merits
are a matter of taste.
A Web recommender may, for example, suggest to a person who has rated
only books about baseball that he might also like a book about ballet.
The recommender would have this information if another person had rated
books on both topics. The recommendation system could unearth this connection
using a nearest neighbor algorithm, which searches for the query point,
or data point nearest the reference.
In this example, the recommendation system, while supplying a form of
advice, has also showed the baseball fan a weak tie, which in social network
theory is a connection between groups that don’t ordinarily interact.
A malicious user could exploit this seemingly incidental piece of information,
according to the researchers.
On the Web, weak ties can be combined with other information to trace
individual users’ identities. Such tracing robs users of the option to
act anonymously, and can be used to mine personal, financial, political
and other information and affiliations.
Even though the risks are intuitively apparent, it's difficult to quantify
the odds of weak tie exposure.
Toward that end, a group of computer scientists from the Virginia Polytechnic
Institute and State University, Purdue University and the University of
Minnesota has analyzed the risks of exposure by mapping the types of connections
users make -- often unconsciously -- when participating in recommendation
systems.
“Our main goal was to quantifiably assess the benefits and risks," said
Naren Ramakrishnan, a professor of computer science at Virginia Tech.
Everybody talks of risks in terms of ‘don't disclose credit card’, ‘don't
disclose age and address’. But we hope to identify more subtle forms of
risk involving seemingly harmless information,” he said.
The researchers did this using graph-theoretic models, which show relationships
and connections among entities in a way similar to family trees, highway
maps and organization charts. By mapping exposure risk, the researchers
quantified the risks and benefits of recommendation systems in general.
“In our case, we use a graph-theoretic model to represent the connections
between people and the artifacts they rate,” Ramakrishnan said. Recommendation
systems make connections between people based on their common recommendations.
Such connections, or jumps, move beyond the common items to the people
who rated them.
These jumps can be represented as social network graphs, which depict
people and how they are related. Recommender graphs go a step further
and include the artifacts, or items that people have rated in common.
With this information, it's possible to find the connection between a
user making a query and one who has rated the item of interest, according
to Ramakrishnan.
Although it’s laborious, a user could game the system and sift for connections
that can be traced back to individuals, said Ramakrishnan. “By varying
the ratings, you might notice that the recommendations change," he said.
"In addition, you might notice that a particular recommendation of book
X happens only for some specific values for ratings. If you know something
about the algorithm behind the recommender system, then you could reverse-engineer
the rating by inspecting the behavior of the algorithm."
To calculate the risk and benefit inherent in a given recommendation system,
the researchers drafted a rough formula: benefit = w/l2, where
w is a connection or connections between people who have rated the same
item or items and l is a sequence of such connections.
"The... higher the w, the higher the benefit. The lower the l, the higher
the benefit. The "squared" is there to make the second statement a little
stronger than the first,” Ramakrishnan explained.
This formula applies to any recommender system that works by making connections,
which is how most of today's e-commerce recommender systems work, said
Ramakrishnan. "Its limitations are that it might have to be adjusted for
individual domains. The formula as it stands is a good qualitative measure,
nevertheless,” he said.
The key is presenting risk in terms of how a person relates to the larger
social context of a recommender system, he said. "Thus, the same person
with the same ratings may not be at risk in a recommender system where
he is just like everybody else; it is his uniqueness [within a given system]
that is posing the risk."
The risk equation can be likened to the way an individual can be singled
out in a crowd, said Ramakrishnan. “If you look like everybody else, nobody
can single you out. If you wear crazy clothes, you will be immediately
spotted. Similarly, if you rate like everybody else, sure you get along
and there is no danger,” he said. “If you rate crazily, on the one hand
you provide a lot of benefit to the recommender, but then you are at risk.”
The researchers are aiming to demonstrate the risks inherent in such rating
systems and broaden the context in which they are considered, said Ramakrishnan.
"We're still studying this area," he said. They are looking into the causes
of weak links, looking for other ways of quantifying benefit and risk
and are looking to derive new ways to manage recommendation systems, he
said.
The use of social network theory to study Web dynamics is compelling,
although the seriousness of these risks is debatable, said David Madigan,
a professor of statistics at Rutgers University.
“Making the connection with the social network literature is fascinating.
[But] is the privacy threat real? I don't think so," Madigan said. The
researchers' example of identifying someone through their ratings seems
"far fetched in the context of large-scale e-commerce,” he said.
A more likely threat comes from old-fashioned violations of privacy agreements,
according to Madigan. “While I might trust, say, amazon.com, a less trustworthy
e-tailer might try my name and password on lots of other sites and get
a complete picture of all the stuff I buy,” he said.
Ramakrishnan’s colleagues were Benjamin J. Keller and Batul J. Mirza of
Virginia Tech, Ananth Y. Grama of Purdue University, and George Karypis
of the University of Minnesota.
Timeline: Now
Funding: University
TRN Categories: Internet
Story Type: News
Related Elements: Technical paper, "When being Weak is Brave:
Privacy Issues in Recommender Systems," posted on the Computing Research
Repository at http://xxx.lanl.gov/abs/cs.CG/0105028
Advertisements:
|
July
25, 2001
Page
One
Sounds attract camera
Interface lets
you point and speak
Quantum logic counts
on geometry
T-shirt technique
turns out flat screens
Rating systems
put privacy at risk
News:
Research News Roundup
Research Watch blog
Features:
View from the High Ground Q&A
How It Works
RSS Feeds:
News | Blog
| Books 
Ad links:
Buy an ad link
Advertisements:
|
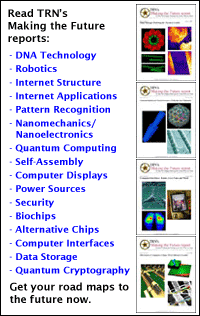
|
|
|