Self-learning
eases quantum computing
By
Eric Smalley,
Technology Research News
Ordinary computers are rather simple devices,
logically speaking. They cannot learn and they have to plow through large
problems one step at a time.
Two largely experimental methods of computing -- neural
networks and quantum
computing -- go beyond these limitations by mimicking biological brains,
and exploiting the quirks of quantum physics, respectively.
A team of researchers at Wichita State University is aiming to combine
the two techniques in order to use neural networks' proven capacity for
learning to help realize quantum computing's potential to solve astronomically
large problems.
The researchers made a simulation of a quantum neural network and used
it to show how the theoretical devices could calculate the quantum mechanical
property of entanglement. Calculating entanglement was an unsolved problem
in field of quantum computing, said Elizabeth Behrman, an associate professor
of physics at Wichita State University
Entanglement is one of the weirder traits of quantum physics. When a subatomic
particle or atom is isolated from the environment and cannot be observed,
it enters into the quantum mechanical state of superposition, meaning
it is in some mixture of all possible states. For example, a particle
can spin in one of two directions, up or down. In superposition, however,
the particle spins in some mixture of both directions at the same time.
When two or more particles in superposition come into contact with each
other, they can become entangled, meaning one or more of their properties,
like spin or polarization, become linked, and move in lockstep. Two entangled
photons could, for example, have linked polarizations. When one of the
photons is knocked out of superposition to become vertically polarized,
the other photon leaves superposition at the same instant and also becomes
vertically polarized, regardless of the distance between them.
Entanglement allows quantum logic operations to work on many particles
at once. A quantum computer can take advantage of entanglement to check
every possible answer to a problem with one series of operations across
a group of entangled particles rather than having to check each possible
answer one at a time.
"Entanglement is the basis for the power of quantum computing," said Behrman.
It is the reason quantum computers are theoretically able to do computations
that cannot be done by even the fastest possible classical computer, she
said.
Quantum computers are extremely delicate, however, and only a few simple
prototypes have been built. And researchers have only come up with a few
algorithms to use them with. Bringing neural networks into the picture
could solve both of these problems, according to Behrman.
Artificial neural networks consist of virtual nerve cells, or neurons,
linked by virtual synapses. Neurons communicate with each other through
the synapses, with the output from one neuron becoming the input to another.
Like biological synapses, the virtual synapses grow stronger with use
and weaken with disuse. Repeated input to a neural network wears a distinct
path through the neurons. In other words, neural networks learn.
"A neural network, like the one between your ears, is different from the
computer on your desk in several important ways," said Behrman.
Because neural networks learn, they can handle incomplete data and scale
up automatically to handle larger problems. "All three of these characteristics
would be great boons to quantum computers," said Behrman.
For example, it is difficult to construct the algorithms, or software
a quantum computer needs to solve a problem, she said. "A quantum
neural computer... essentially constructs its own algorithm," she said.
The entanglement calculation demonstrates that quantum neural networks
should be able to work for any of the many difficult problems that researchers
are building quantum computers to solve, said Behrman. "We don't need
to construct an algorithm for each of them if we have a quantum neural
network," she said.
The ability of neural networks to handle incomplete data could be helpful
because quantum computers are very sensitive to noise, said Behrman. "We're
working on showing that neural computers can help with this [problem],
too, but we haven't yet demonstrated it," she said.
Quantum neural networks could also help make larger quantum computers.
"The advantages of scale-up are obvious," said Behrman. "At the moment,
we have quantum computers that are only of the size of a few qubits."
Quantifying entanglement is a very important question in quantum information
theory, said Vlatko Vedral, a lecturer of physics at Imperial College
and Oxford University in England. "Entanglement is at the root of quantum
teleportation, quantum cryptography and some quantum algorithms," he said.
Having a computer that learns to compute an entanglement algorithm is
useful because the algorithm "involves an optimization procedure
that is difficult to perform," he said.
The idea of using a quantum neural network to compute entanglement needs
to be more thoroughly explored to determine if it offers any advantages,
however, said Vedral. "One thing that is not convincing in the [researchers']
paper is that their method is efficient," he said.
The Wichita State University researchers are beginning a collaboration
with other researchers to attempt to build a quantum neural network, said
Behrman. Quantum neural networks could be used in practical applications
within 10 years, she said.
Behrman's research colleagues were Vishwas Chandreshekar, Zhonghua Wang,
Chaitra Belar, James Steck and Steven Skinner of Wichita State University.
The research was funded by the National Science Foundation (NSF).
Timeline: <10 years
Funding: Government
TRN Categories: Quantum Computing and Communications; Neural
Networks
Story Type: News
Related Elements: Technical paper, "A Quantum Neural Network
Computes Entanglement," posted on the arXive physics archive at http://arXiv.org/abs/quant-ph/0202131
Advertisements:
|
July
10/17, 2002
Page
One
Photons heft more data
Eavesdropping gets
people talking
Self-learning
eases quantum programming
Conceptual links
trump hyperlinks
Cell parts paint picture
News:
Research News Roundup
Research Watch blog
Features:
View from the High Ground Q&A
How It Works
RSS Feeds:
News | Blog
| Books 
Ad links:
Buy an ad link
Advertisements:
|
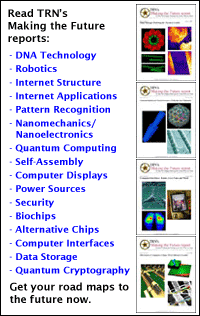
|
|
|